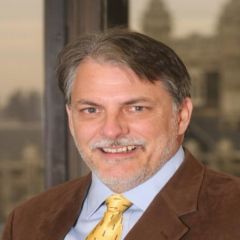
Dr. Kyle McDonald
Dr. Kyle McDonald is the Terry Elkes Professor in the Department of Earth and Atmospheric Sciences at The City College of New York. A leading expert in microwave remote sensing, he specializes in electromagnetic scattering and propagation, with a focus on monitoring terrestrial ecosystems and the cryosphere. His research integrates satellite observations with ecosystem and hydrologic models to better understand land-atmosphere carbon and methane fluxes, surface hydrology, and vegetation productivity—particularly in cold regions.
Dr. McDonald has led extensive work using active and passive microwave sensors to interpret and model backscatter and emissivity signals in complex land surfaces. His primary focus in recent years has been on seasonal dynamics in boreal ecosystems and their relation to ecological and hydrological processes. He is widely recognized for his contributions to the use of remote sensing in cold land ecology and hydrology.
His research activities include designing and conducting field experiments, developing ground-based instrumentation, collecting and interpreting field and satellite data, and modeling radar signals. He has utilized a wide range of satellite and airborne platforms including ERS-1/2, JERS-1, RADARSAT, ALOS, NSCAT, SeaWinds, AIRSAR, UAVSAR, AMSR-E, and SSM/I.
Dr. McDonald has played key roles in advancing several NASA and ESA Earth mission concepts such as NISAR, SMAP, Hydros, and CoReH2O. He served as the lead scientist for the freeze/thaw element of the Hydros mission and contributed significantly to its selection for NASA’s ESSP-3 program. He also supported the SMAP mission in related algorithm development.
He is a member of NASA’s High Mountain Asia Team (HiMAT), the NISAR Science Team, and the Japanese Space Agency (JAXA) Kyoto and Carbon Initiative, contributing to global research on carbon cycle dynamics using PALSAR datasets. His current work continues to explore the link between vegetation biophysical properties and radar backscatter, combining remote sensing with process-based modeling to improve environmental monitoring at multiple scales.